Jan. 27: Invited Speaker Katie Keith to Present on Proximal Causal Inference with Text Data
<h3>One-U Responsible AI faculty fellow Ana Marasovic will host Keith</h3>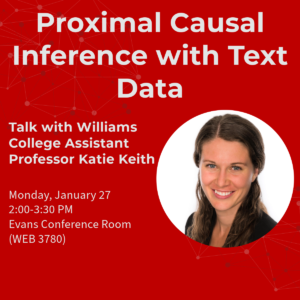
Speaker: Katherine (Katie) Keith, Assistant Professor in the Computer Science department at Williams College
When: Monday, Jan. 27, 2025, 2–3:30 pm
Where: Evans Conference Room (WEB 3780), Warnock Engineering Building, University of Utah, 72 S Central Campus Dr, Salt Lake City, UT 84112
Proximal Causal Inference with Text Data
Abstract
Causal inference underlies many important policy decisions and interventions. For example, clinicians must decide how to prescribe medications to patients, central bank committees must decide how to change interest rates, and online platform administrators must decide how to moderate users. In the absence of a randomized controlled trial, one can turn to observational (non-experimental) data to estimate causal effects. In this setting, a primary obstacle to unbiased causal effect estimation is confounding variables, variables that affect both the treatment (e.g., which medication) and the outcome (e.g., an aspect of patient health). In many applications, a rich, unstructured source of confounding variables is text data: notes from electronic health records (EHRs) detail patients’ personal and medical histories, newspaper articles document national and international events, and online platforms host exchanges of users’ written opinions. By expanding observational causal estimation methods that can incorporate natural language processing (NLP) and text data, analysts may be able to make inferences in a wider range of settings.
Recent text-based causal methods attempt to mitigate confounding bias by estimating proxies of confounding variables that are partially or imperfectly measured from unstructured text data. These approaches, however, assume analysts have supervised labels of the confounders given text for a subset of instances, a constraint that is sometimes infeasible due to data privacy or annotation costs. In this work, we address settings in which an important confounding variable is completely unobserved. We propose a new causal inference method that uses two instances of pre-treatment text data, infers two proxies using two zero-shot models on the separate instances, and applies these proxies in the proximal g-formula. We prove, under certain assumptions about the instances of text and accuracy of the zero-shot predictions, that our method of inferring text-based proxies satisfies identification conditions of the proximal g-formula while other seemingly reasonable proposals do not. To address untestable assumptions associated with our method and the proximal g-formula, we further propose an odds ratio falsification heuristic that flags when to proceed with downstream effect estimation using the inferred proxies. We evaluate our method in synthetic and semi-synthetic settings—the latter with real-world clinical notes from MIMIC-III and open large language models for zero-shot prediction—and find that our method produces estimates with low bias. We believe that this text-based design of proxies allows for the use of proximal causal inference in a wider range of scenarios, particularly those for which obtaining suitable proxies from structured data is difficult.
Biography
Katherine (Katie) Keith is currently an Assistant Professor in the Computer Science department at Williams College in Massachusetts. Her research interests are at the intersection of natural language processing, causal inference, and computational social science. Previously, she was a Postdoctoral Young Investigator at the Allen Institute for Artificial Intelligence, and she graduated with a PhD from the Manning College of Information and Computer Sciences at the University of Massachusetts Amherst. She has been a co-organizer of the First Workshop on Causal Inference and NLP, co-organizer of the NLP+CSS Workshops at EMNLP at NAACL, and was a recipient of a Bloomberg Data Science PhD fellowship.